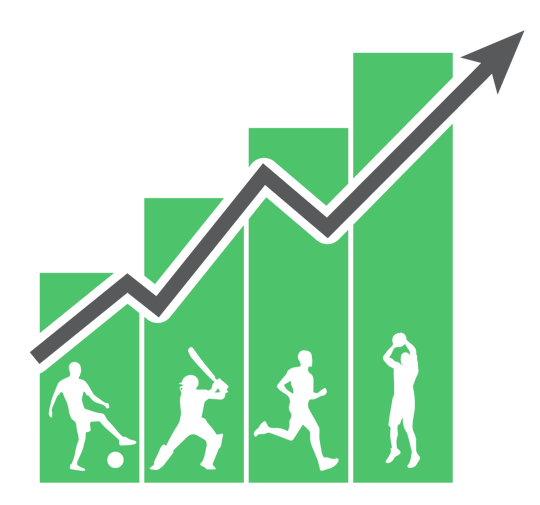
Sports Data Club
Using data in Sports
EN
I am a Data analytics professional with a passion for sports.
I will use this website as a portfolio and will try to show things that are not "out there" in the sports world and media. In this space you will be able to see some examples and interact with them. **Visit the last Blog entry ** where I explain how we are now working with Baxi Manresa! (ACB Spain)
ES
Soy un profesional en el campo del Data Analytics con pasión por los deportes y con ganas de mostrar datos, visualizaciones... que no están a la vista diariamente. En este espacio podréis ver ejemplos de mis trabajos e interactuar con ellos. **Lee la última entrada al blog ** donde explico como ahora trabajamos con Baxi Manresa! (ACB)
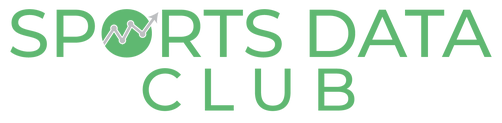
*Example of Player Profile Card (Small version)
Portfolio
I´ve been working on several types of visualizations within football, basketball and other sports, mainly to websites like Baskeroseno and Smart Scouting Club.
These dataviz could contain different metric, some simples ones as goals, passes, points, possessions... and other more advance statistics like xG (Expected goals), USG, ORTG, etc.I represent these on multiple styles like XY graphs, Radars, or more complex visualizations like Sankey Diagrams.I use (among others) Tableau software to plot these stats.In this website you can play around with some of my examples. Click the links at the right to see the examples from my personal portfolio (If you see some size errors in the visualizations, please press F5 to refresh).Also, please be aware that to enjoy the full interactive viz, is better to visualize them on Desktop/Tablet.You can also check my Interactive CV, my Tableau Public profile and other examples in the Blog space.Any question, please feel free to reach me on the contact section!
Radar Chart
One of the most common dataviz to compare players and teams. In a quick view, it gives you an understanding of who is doing better and in which fields.
You can see here and below 2 examples. At the left, comparing 2 football players.
Other option is using Python to create the Radar chart:
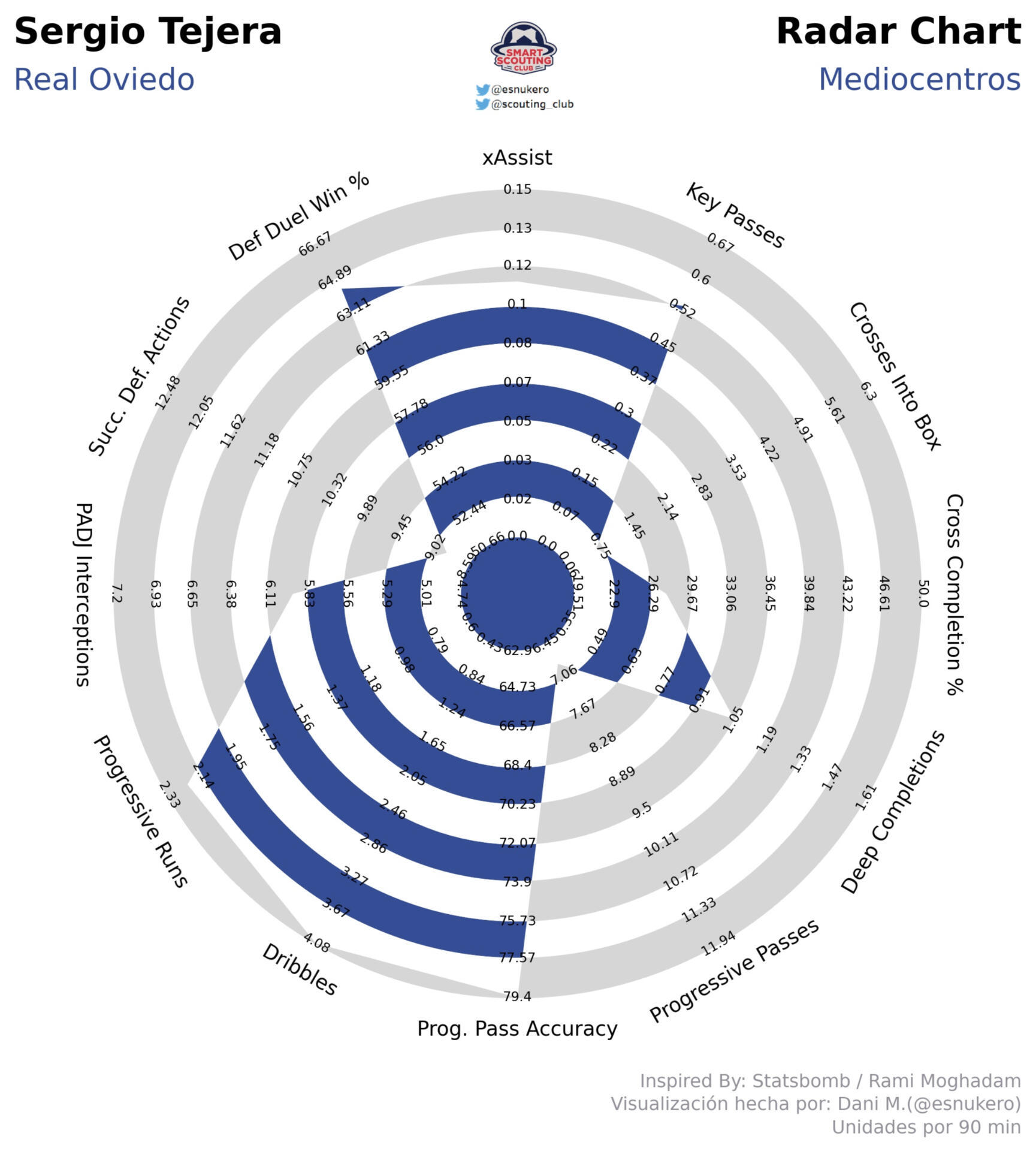
Here it is another Radar example, this time for the Basket Euroleague comparing 2 teams.
Network Passes
Made with Python, this viz shows all the average position of the player (giving or receiving successful passes) and their connections and amount of passes.
This viz is really useful to show styles of game in the distinct parts of the game.
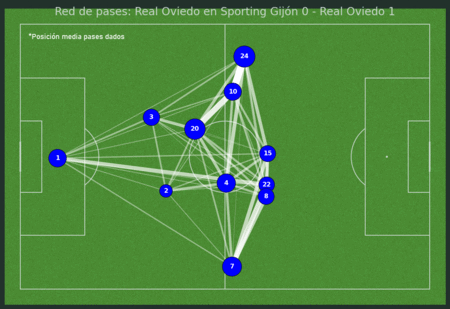
Player Profile in the League
This useful view gives a clear vision of how is the player or team performing.
First image shows the actual numbers of Harry Maguire compared to the Top 6 League Central Defenders, showing Min, Average and Max actual numbers. Also it shows a "Percentil Quadrant" vs the rest of the data group.
In the second image, the length of the bars show the position in the percentile of the league, and the number shows the actual value of that stat.
The third example shows the Percentile % in the League of the player and the actual value of the stat.
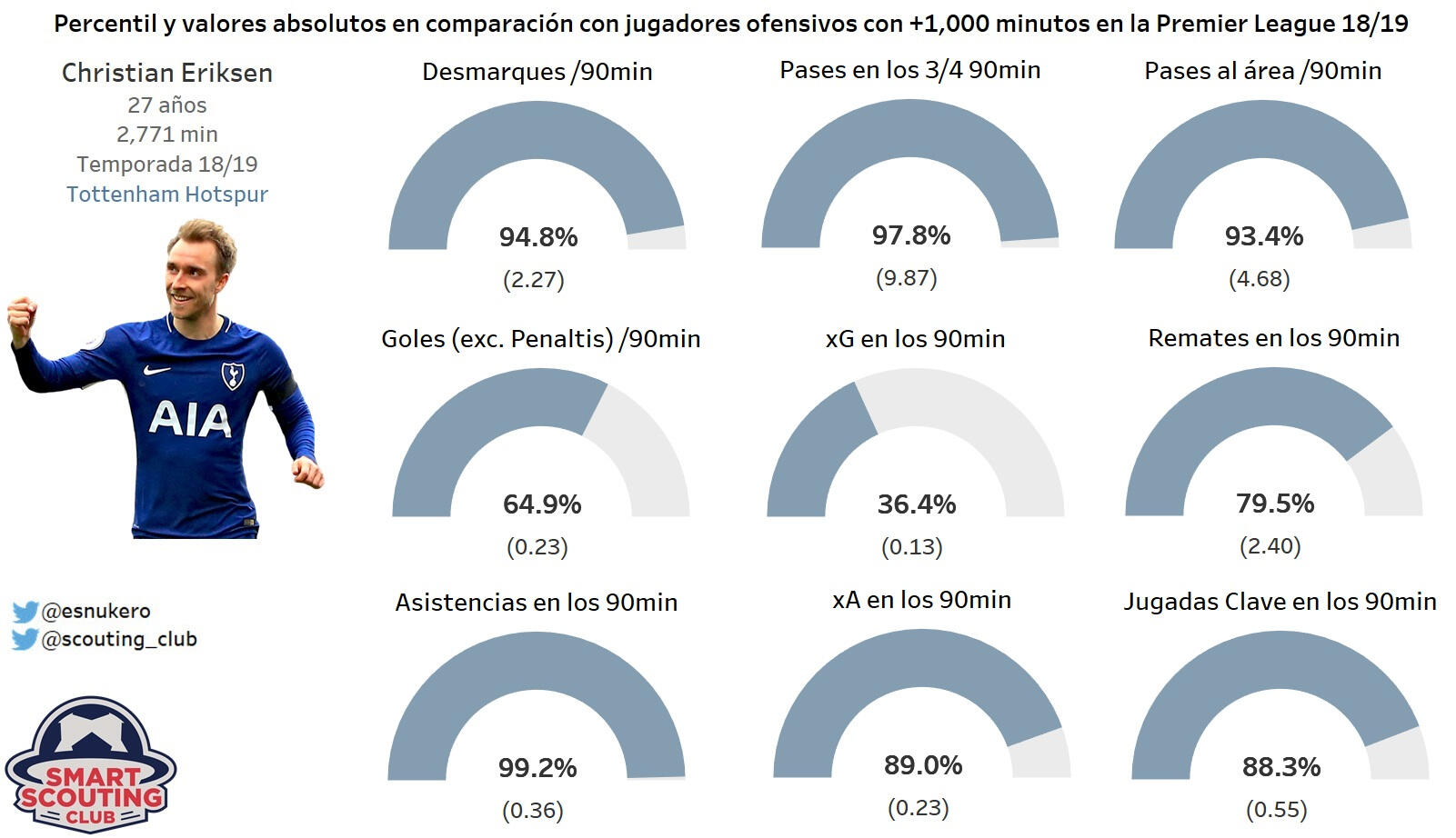
Player Comparison / Finder
Usually we are curious to see which players could replace one of our players. This tool wants to show that.In this example with the Second Division in Spain, we see how in the first 15 games, Adri Embarba "most similar player" was Isi Palazón according to the tool.
Few weeks later, Embarba left Rayo Vallecano to play in the First Division with Espanyol and they signed Isi from Ponferradina. Coincidence? :)Please feel free to reach if you want to try it.
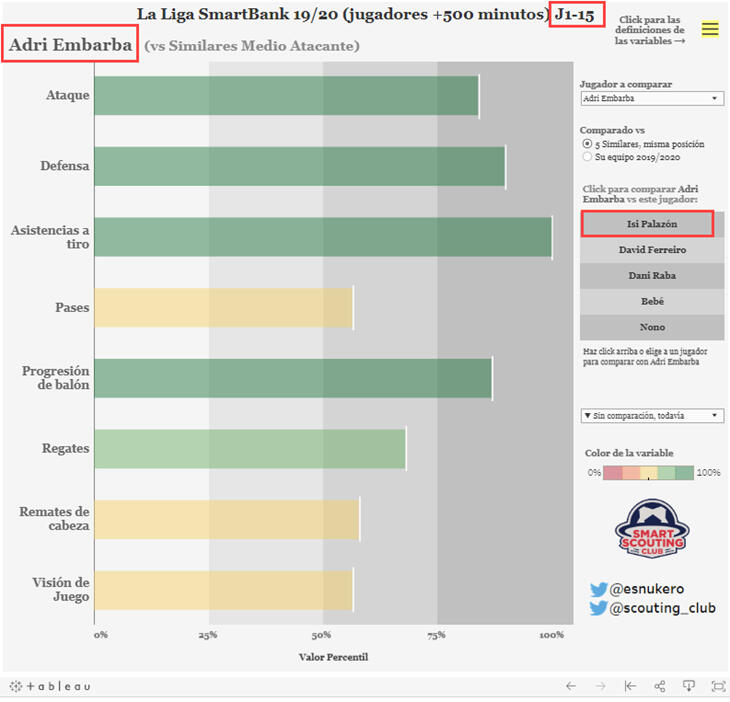
Player Actions Dashboard
I like to name this the "Control Panel" of actions for players or teams.
Here you can select among almost 100 metrics of a team (Real Oviedo in Segunda League Spain) on X and Y Axes. This view makes easy to visualize who where the best/worst players on certain stats of the game.
Expected Goals (xG) For and Against
xG (Expected goals) can give us a different view on how were the occasions created by a team or player. Here we can see the trend from some teams of the Japanese League, if they are over or under performing.
XI Line up - Stats view
In this view we can show the most used XI of the team plus all the possible available stats to check per position. Just hover over each point to see the different players stats. The size of the point shows you the total sum of that stat selected.
La Liga 18/19: NP Goals - NP xG / 90min vs NP Goals Scored/ 90min
Below you can check how La Liga players performed this season, if they over or under performed on occasions created and goals scored. You can filter by team, minutes played and highlight by their position.
You can see all the Top 6 European Leagues Players on this blog entry
La Liga Segunda 19/20: Minutes played vs Age of the players
Below you can check how Second League in Spain players performed this 19/20 season, compared with their age and minutes played by position.
Expected Goals (xG) Trends
A good model of Expected Goals (xG) can show how is performing a certain team attacking and defending. In the example below you can see the Japanese League, showing xG For and Against. Top right of the graph would show the teams that have more contributions that are expected to be a goal, and also that concede less opportunities.
xG Contributions
Using xG (Expected Goals) and xA (Expected Assists), we can have a view of xG contributions, that will give us the players who could be the most decisive in the attacking part during the season. 2 examples below for La Liga and La Liga123.
*If browsing on the phone, please swich to Landscape view.
xG Timelines
Another way to show Expected Goals (xG) is through the timeline of a match, where in a quick look we can check how dangerous were the shots for both teams. Below and example from the Japanese first division.

Other possible view is the one below, by action and minute, where you can click the bar and it will give you info in the box about that action (who was the player, minute, xG value, if it was goal or not...)
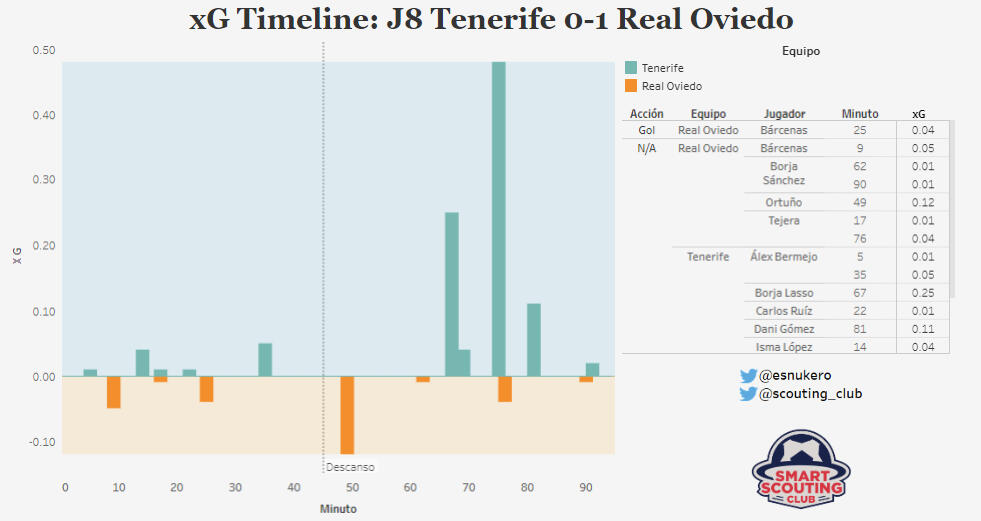
XY Axis visualizations
Two dimension graphs are used a lot in several sports to show teams or players under 2 stats. It´s easy to check where they perfom good or not so good.
In this examples we see the ACB Teams per PPP and Poss/Game in the last 2 seasons pe type of attack, and Fenerbahce Players prior to the Euroleague Basketball Final Four. Graph shows % Time vs PPP in Spot up game type.
Another XY example, this time NBA. In the Y axis we see the rank of a team on certain stat which appears on the X axis.
Parallel Coordinates Plot
This type of visualisation is used for plotting multivariate, numerical data. Parallel Coordinates Plots are ideal for comparing many variables together and seeing the relationships between them.
Below, we compare some of the variables from the strikers of Segunda División in Spain.
symmetric axis viz
This symmetric axis visualization shows the minutes played per age of the players from the different teams of the Second Division League in Spain. The higher and wider means that older players played more minutes. You can check the viz and see the minutes per age hovering it here.
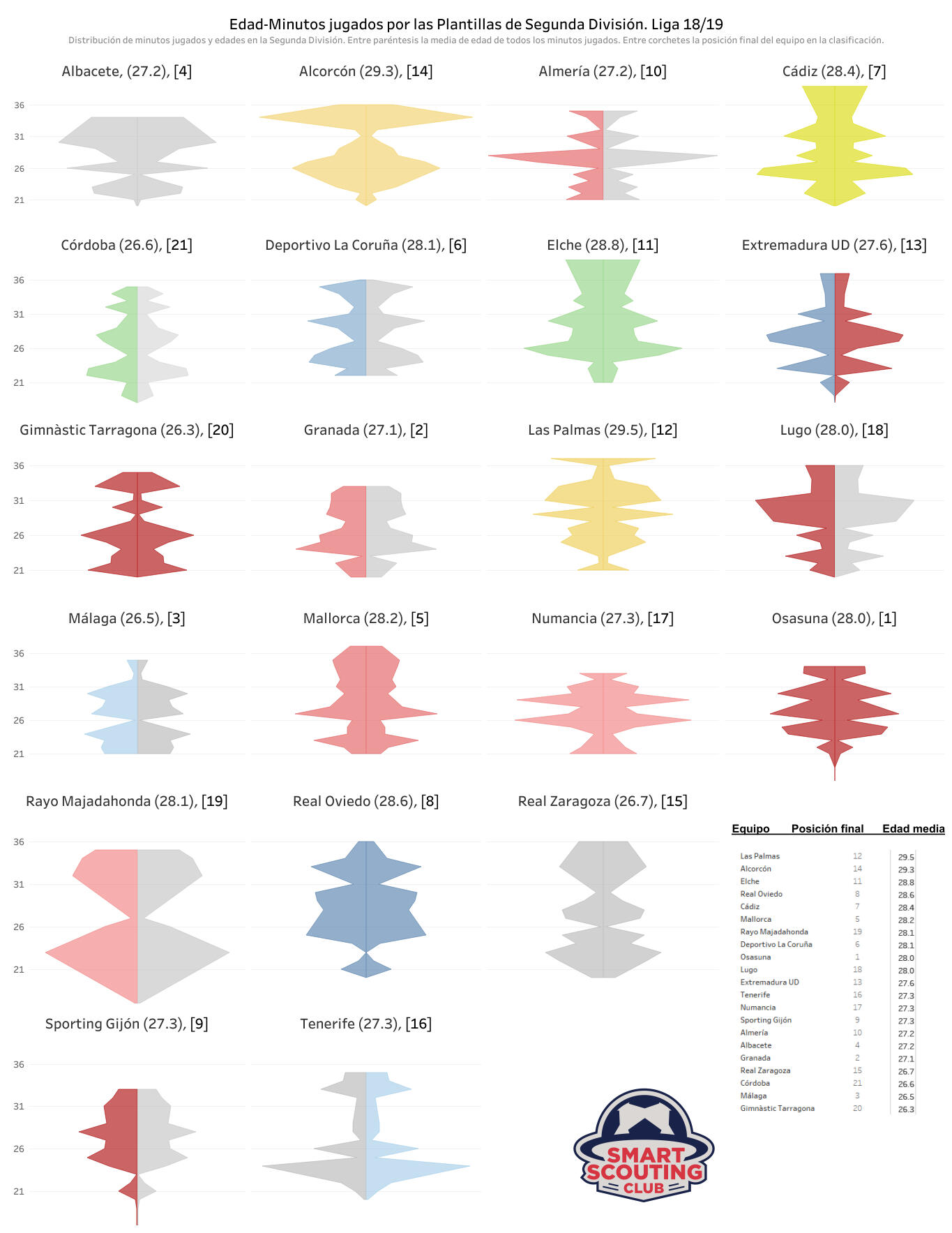
Jitter plot (2 dimensions)
The main reason to use a Jitter plot rather than a strip plot is when you have too many marks overlapping and you want to be able to select any individual mark (which is difficult or even impossible if the marks overlap entirely). Below you see this plot but applied to 2 dimensions in an Euroleague example with the Spanish team, where number of shots and efficacy are related. There are 2 tabs to choose different views.
Pass and Actions map
Focusing on one player and showing all his key actions in a match and where occurred, usually used for goals. Also the "pass map" shows the most common passes to other players on the pitch. First, you have a complete dashboard with "eventing" actions from a team in a pitch.
"Sankey" Pass Diagram
This colorful viz is useful to see how is the pass flow of an specific player. Which is the most common past he does? and from which player he receives more passes? You can see it below depending on how thick is the line.
"Sunburst" Diagram
A mixture of a pie chart and a donut chart, the Sunburst diagram is useful to represent categories and subcategories. This example is about field shots of a basketball player, made and attempted from different play types.
Play Types: Volume & Efficiency. Card Style
This is a Card Style viz, used for Basketball Play Types. Here you can compare Volume and Efficiency of 2 players in all the Play Types.
Points per Possessions (PPP)
The PPP metric is one of the most used on Basketball on these days.
Here you can see some ways to present it in a clearer way to your audience if they are not really into numbers.
Image 1: Comparison between teams and types of plays PPP
Image 2: Individual Stats per team and players
Image 3: PPP Team and Type Timeline comparison
Image 4: Season PPP comparison per Type
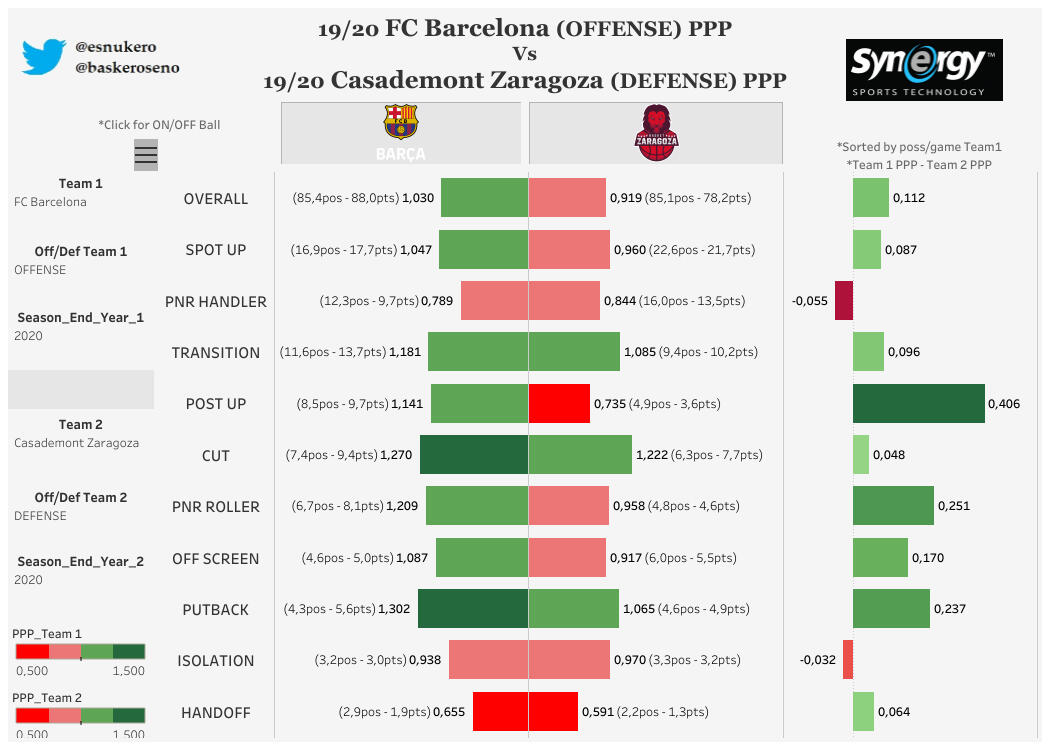
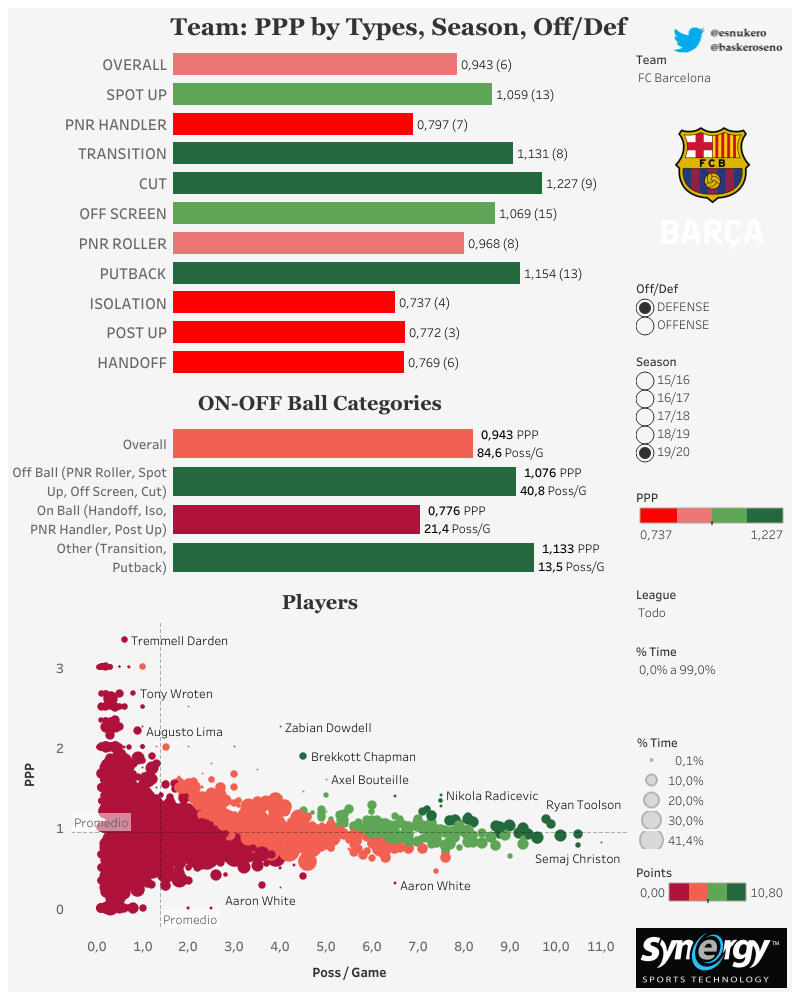
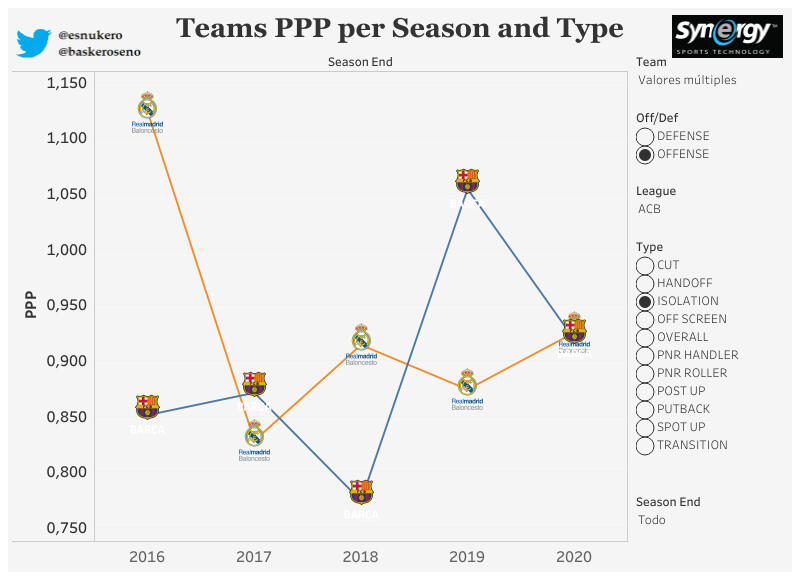
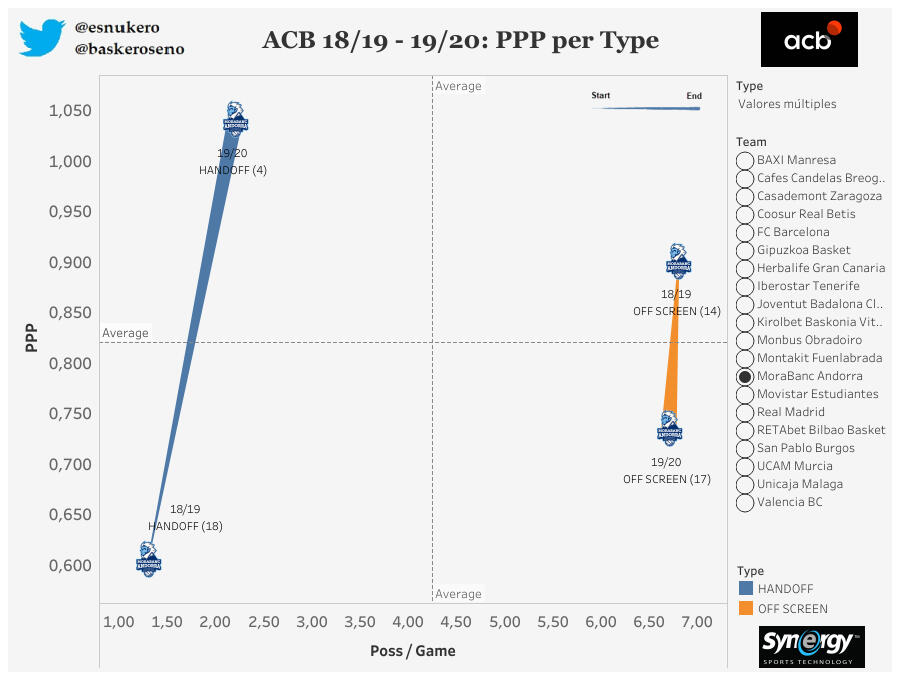
Comparison Tool and PPP Team Profile Dashboard
Here you can see two example of panels. The firs one contains a sample of a PPP comparison tool per teams, useful to study differences between teams. There is the same available for Players.
The second one is a "one team" panel dashboard to check individual performance and how are those numbers in the team and vs last season.
Basketball Line Ups
In this graph, it shows all the data available for Line Ups from the Spanish Basketball Cup.
Several filters to select teams, minutes played, metrics on X and Y axis, look for a player... and below a ranking for certain metric.
This is great to check which line ups are more efficient.
Clustering
Nowadays is common to use the clustering method to group players or teams with the same numbers or qualities.
In this example I show the Progression on the Player Impact Plus Minus (PIPM) with 4 clusters of NBA players along these past 10 years.
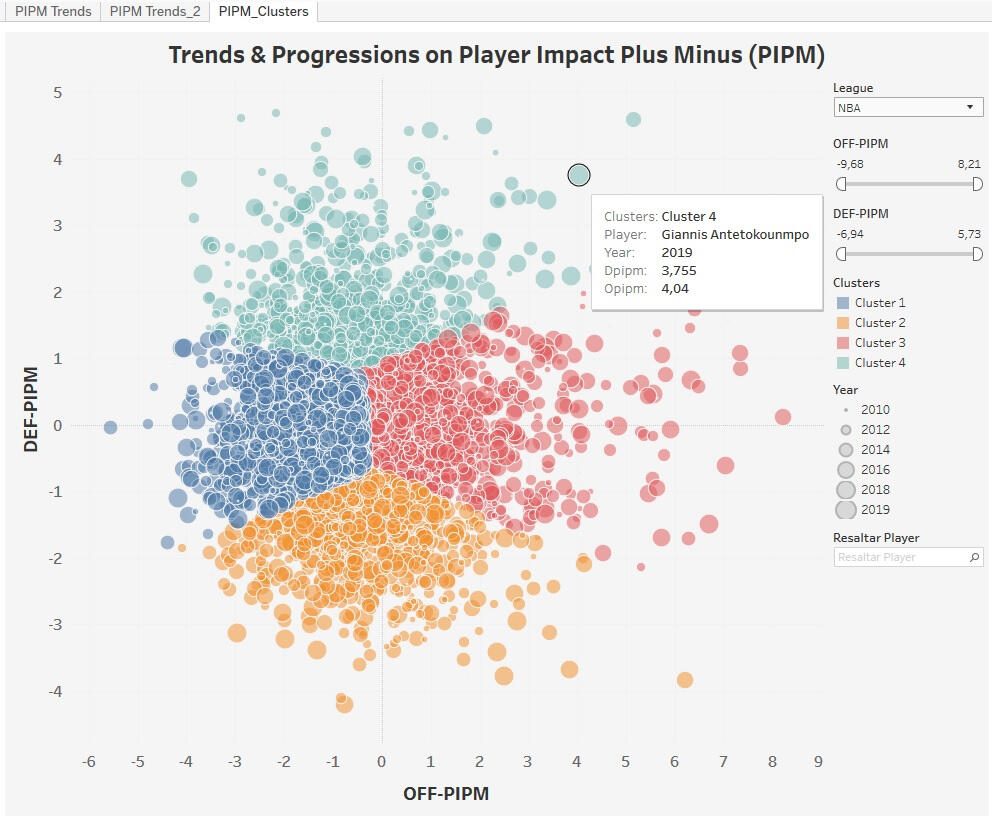
Bar Charte Race viz
One trendy viz that shows in a nice way a trend per time. Below you can see one about the top goalscorers of my team, Real Oviedo, over the years, and the % of snooker players for each nationality per season.
Dynamic Dashboards with several filters
This football example shows the dribbles per 90 min and the successful ones in the Premier League. You can filter by team, position, minutes played...
*If browsing on the phone, please swich to Landscape view.
Also useful for Basketball examples. Here we can see the different Possessions types and the volume & Efficiency per player in the Euroleague.
Visual Dashboards
It´s always nice to offer information in a different visual way. Here you can see a flower style dashboard with some of the triple crown winners on Snooker (a billiard modality which I follow).
Below you can see a Career Dashboard of a Snooker Legend, Ronnie O´Sullivan.
*If browsing on the phone, please swich to Landscape view. Better to see on Desktop.
Predictive applications
You can apply visualizations to predictive models. Below, you can see a graph where I applied a predictive model for the Segunda División table. From fixture 17, you can move fixture by fixture until the end of the season.
*If browsing on the phone, please swich to Landscape view. Better to see on Desktop.
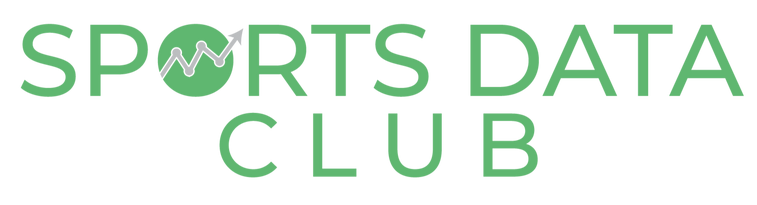
About me - ¿Quién soy?
EN
My name is Daniel Martínez, originally the north of Spain, I finished my Master Degree on Chemical Engineering and in these years I developed a professional career on Data Analytics in industries like Gambling (London, Gibraltar) and Online Marketing (Barcelona).
I finished a University Course (Valladolid University) to become "Expert in Sports Data Analytics", using data and tools like: Opta Sports, iSF, Tracab, MediaCoach, NAC Sports, STATs, Wyscout, InStat and ERiC.
Right now, I work as a Data Product Manager in the manufacturing section of an e-commerce global company.ES
Mi nombre es Daniel Martínez, soy de Oviedo, Asturias.
Licenciado en Ingeniería Química, mi vida profesional siempre ha estado ligada al mundo del análisis y los datos, reporting y visualizaciones (en industria del Juego Online y Marketing Online, Londres, Gibraltar y Barcelona).
En estos últimos años he reforzado mi curiosidad en el mundo del "Sport Analytics" y he realizado el curso de "Experto en Análisis de Datos y Big Data" de la uVa (Universidad de Valladolid).
Ahora mismo trabajo como Data Product Manager en la sección de Manufacturing en una empresa de e-commerce global.
Debajo puedes ver un CV interactivo con información anual al pasar el ratón o clickar encima de cada punto.
CV Interactivo (en cada punto verás más información)
LInks to latest articles
*If browsing on the phone, please swich to Landscape view. Better to see on Desktop.
Votación para el Mejor Defensor en Movistar #Vamos
Esta semana, Ander de Baskeroseno ha participado en la votación del mejor defensor de la Liga Endesa ACB 21/22 en el programa de Movistar #Vamos.Dentro de este video con la votación, se introdujeron un par de gráficas para evaluar el poder defensivo del Manresa en la Liga y también para poner en contexto de manera fácil y visual para gente que no está acostumbrada a los datos la actuación de Chima Moneke en varios aspectos defensivos.Aquí podéis ver el video de la participación de Ander.
Colaborando con Baxi Manresa
Ha pasado un tiempo desde la última actualización del Blog. Entre medias hemos vuelto a colaborar con la Universidad de Mondragon en su grado de Business Analytics y también pudimos anunciar que hemos empezado a trabajar codo con codo con el Baxi Manresa de la Liga ACB de Baloncesto en España.
Esta colaboración se basa en el análisis de datos y herramientas de visualización que desde Baskeroseno hacemos para que el staff técnico optimice el proceso de scouting y la toma de decisión.Hablaré más sobre esto en próximas entradas al blog!
Derby Asturiano
Aquí podéis ver ne modo gráfico la historia de todos los Derbies Asturianos entre el Real Oviedo y el Real Sporting a lo largo de los años. Cada punto es un partido, pasa el ratón por encima para ver el resultado y más información (Recomendable ver en pantalla de ordenador).
Link al artículo: https://www.lavozdeasturias.es/noticia/azulcarbayon/2021/04/17/derbi-late-azul/00031618614363476791755.htm
DATOS & BALONCESTO: Reto con Baskonia y Mondragon Unibertsitatea (Grado en Business Data Analytics)
Hace unas semanas estuvo mi compañero Ander Isuskiza (Baskeroseno.com) en la nueva sede de Mondragon Unibertsitatea para impartir un par de sesiones en el reto con Baskonia - Alavés Group que deberán superar los alumnos del Grado en Business Data Analytics.El objetivo era acercar el uso de estadísticas avanzadas en el baloncesto profesional y cómo gestionar las bases de datos para detectar perfiles similares a jugadores ya conocidos y aprovechar oportunidades de mercado.Aquí podéis ver un video resumen del proyecto.
Cómo usar estadísticas para jugar y fichar mejor" (Debate Sindicato de Entrenadores de Baloncesto)
Durante este periodo de confinamiento por la COVID-19, el Sindicato de Entrenadores de Baloncesto (@SinebDe) está llevando una iniciativa de realizar diversos debates sobre temas que afectan al Baloncesto y sus entrenadores.Encargaron a mi compañero Ander Isuskiza (Baskeroseno.com) el capítulo de Estadística Avanzada dentro del Baloncesto, su aplicación en los aspectos tácticos del juego y de dirección deportiva y cómo administrar y presentar esos datos a los entrenadores y jugadores para que les sean más fáciles de entender.Yo he participado en la parte gráfica, con las visualizaciones de Tableau. Los dashboards o "paneles" son una buena herramienta de plasmar estos números en algo visual que los entrenadores y jugadores puedan "digerir" mejor y con ellos llegar esas ideas o percepciones con los que los números pueden responder algunas cuestiones que se plantean los entrenadores.Os animo a ver el vídeo de la presentación (unos 20 minutos) en el que se tocan varios puntos que empiezan a sonar cada vez más en el baloncesto europeo y que sin duda seguirán creciendo en los próximos años.
Colaboración en La Voz de Asturias
Aquí podéis leer una un artículo sobre el Real Oviedo en el periódico La Voz de Asturias que se complementa con uno de mis gráficos.
Entrevista en diario Futbol Asturiano
Aquí podéis leer una entrevista que me ha hecho Guillermo Bañó para el periódico digital Futbol Asturiano
Análisis de jugadores de baloncesto a nivel de scouting usando datos (I)
En el Baloncesto, los datos ya forman parte del día a día de muchos equipos Americanos, y en Europa y el resto del mundo poco a poco se empieza a ver como se usan los datos activamente para preparar partidos o para scouting.En el último año he estado trabajando con Ander Isuskiza de Baskeroseno.com en maneras de explotar y visualizar estas métricas a las que él tiene acceso. En su web podréis ver numerosos análisis con algunas otras visualizaciones y datos.Desde el lado de vista técnico (en la parte de baloncesto no puedo ayudar mucho...) los datos que se pueden descargar hay que tratarlos, ordenarlos y limpiarlos antes de cargarlos a un programa para visualizar que en este caso es Tableau. Hacer una ETL con la idea de responder: ¿Para qué quiero estos datos?, eliminar duplicados, crear nuevas métricas combinando las existentes (necesito algo por x minutos jugados?), para dar al usuario final un panel self service para que filtre y seleccione a su gusto.El panel mostrará a los jugadores por percentiles, como modo de filtro antes de realizar un análisis más amplio y ver sus números actuales.El objetivo de este panel es poner en perspectiva a todos los jugadores de una liga (o conjunto de ligas) para comparar rendimientos en diferentes aspectos del juego.
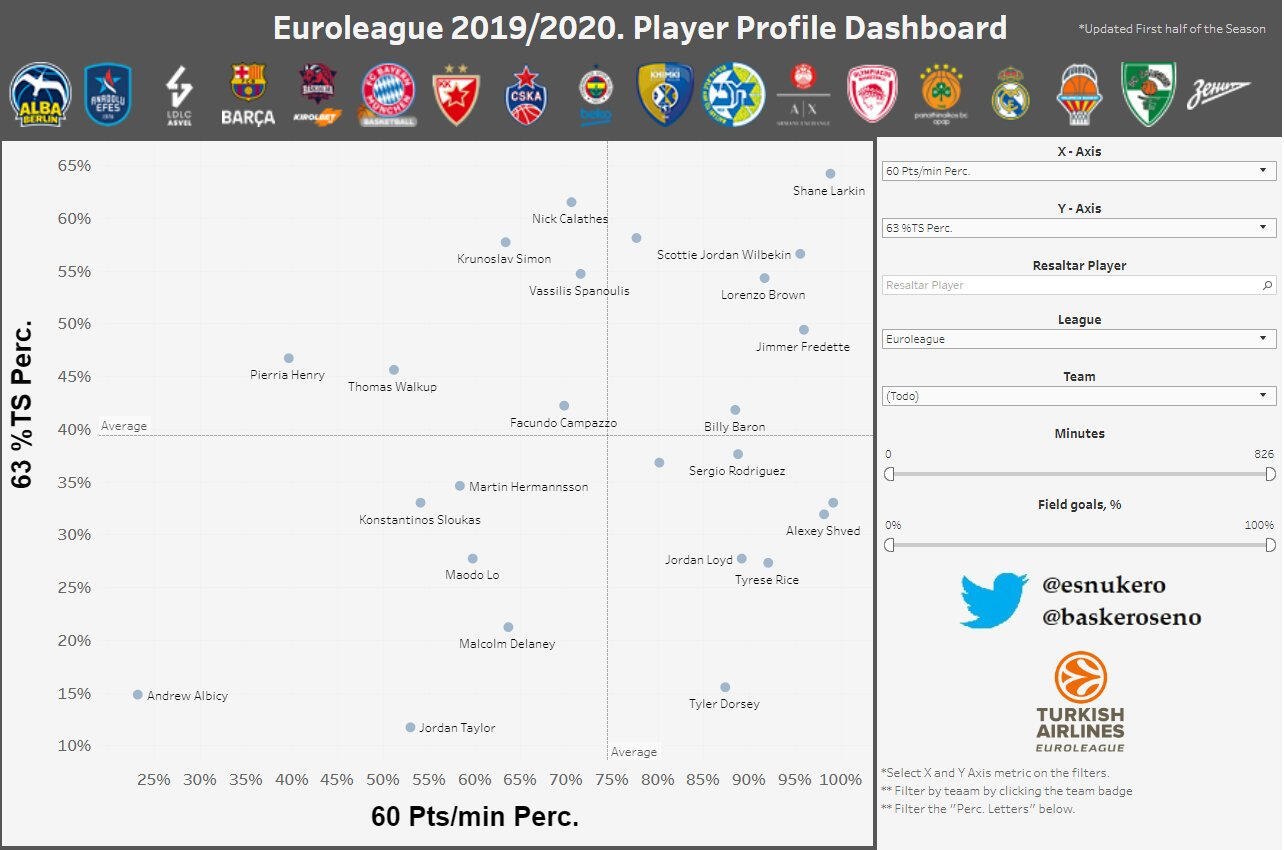
Para ello, utilizamos 85 filtros que definen, a base de percentiles, dónde se sitúa cada jugador tanto en métrica más convencionales (puntos, rebotes, asistencias) como compartimentando más el juego (tiros tras bloqueo, en contraataque, etc).
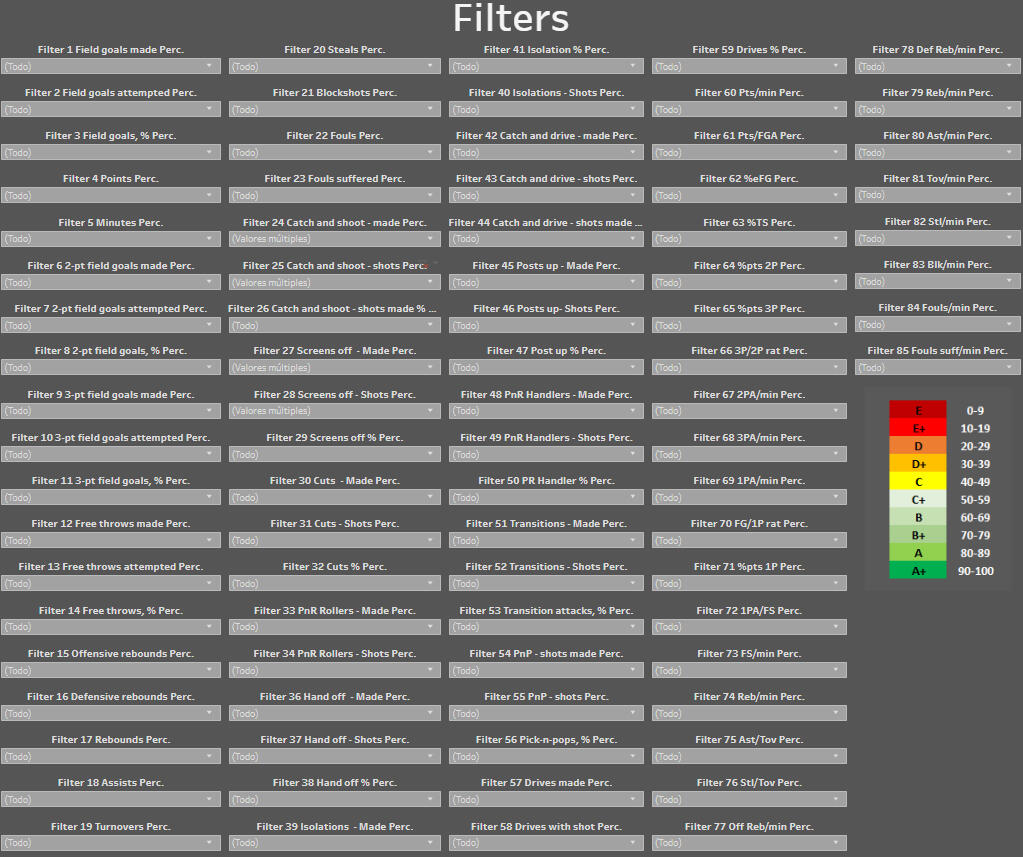
Además, los percentiles se transforman en letras de la A (90-100) a la E (0-9), lo que permite comprender mejor la calificación en cada apartado.A su vez, al pinchar en un escudo podemos ver los jugadores de un equipo destacados sobre el resto de la muestra, lo que nos puede ayudar a contextualizar su nivel.

Un panel de estas características nos permite hacer un análisis profundo en dos niveles de scouting: optimización a nivel táctico y detección de talento.El primero busca encontrar en qué situaciones ha sido más o menos efectivo el jugador y se está explotando lo suficiente o demasiado ese tipo de jugada. Igualmente, permite saber si hay que optar por dar menos minutos al titular y más al suplente en caso de que el rendimiento así lo sugiera. De todas formas, hay que tener en cuenta que, generalmente, con un mayor volumen de tiros es difícil mantener una efectividad alta.Por otro lado, a nivel de buscar jugadores para fichar, esta herramienta permite encontrar jugadores de perfiles similares en ciertas situaciones del juego. De esta manera, identificamos un "molde" y encontramos los que se parecen pero tienen menos estatus o un salario inferior, por ejemplo, y así se convierte en un objetivo de fichaje.

El siguiente objetivo es incrustar estas últimas tablas en el panel para que se puedan ver los números de un jugador en cada tipo de jugada al clicar sobre él o buscarlo.Para los aficionados al baloncestos, ¿Qué opináis? ¿Veis valor en estas ayudas de cara a planificar partidos e incluso para mejorar plantillas de cara a fichar nuevas caras? Sin duda creo que estos análisis serán más y más habituales y la figura del analista de datos irá totalmente de la mano con la del analista del equipo/jugadas.
Entrevista a blog externo
Aquí podéis leer una entrevista que me han hecho de 11 preguntas que han hecho a multitud de personas relacionadas con el fútbol y analytics. (En inglés)
https://undervalu9.blogspot.com/2020/01/11-questions-with-esnukero.html
Comparador de Jugadores basado en datos 19/20
Actualmente existen varias compañías que ofrecen métricas de jugadores y equipos para gran cantidad de partidos de fútbol ya jugados.
Estas métricas como pueden ser los tiros a puerta, regates, pases de diferentes clases, centros, acciones defensivas, saltos, la eficacia en cada uno de ellos… pueden ser muy interesantes y útiles pero muchas veces no ofrecen una descripción del jugador sólo viendo estos valores por separado.
Por eso, una opción es unir estas métricas en una “métrica general” como puede ser Ataque, Defensa, Pases, Asistencias… y poder crear una imagen global de un jugador comparándolo con el resto de los jugadores de su posición.
Para este “panel de comparación” he utilizado 8 métricas generales, que han sido generadas con unas 50 métricas de acciones individuales que ofrecen las compañías (en este caso Wyscout)
Estas métricas generales son: Ataque, Defensa, Pases, Progresión de balón, Asistencias a tiro, Regates, Remate de Cabeza y Visión de juego.
Estos grupos se han aplicado a los jugadores (más de 200 minutos jugados en esta liga, por ahora sólo 11 partidos) divididos por posiciones: Defensa Central, Defensa Lateral, Mediocentro no ofensivo (defensivos y creadores), Mediocentro ofensivo (Extremos y medias puntas) y Delanteros.
En este ejemplo se muestran los números del Panel de comparación (Tableau) del joven noruego de 20 años, Martin Ødegaard, cedido por el Real Madrid a La Real Sociedad.
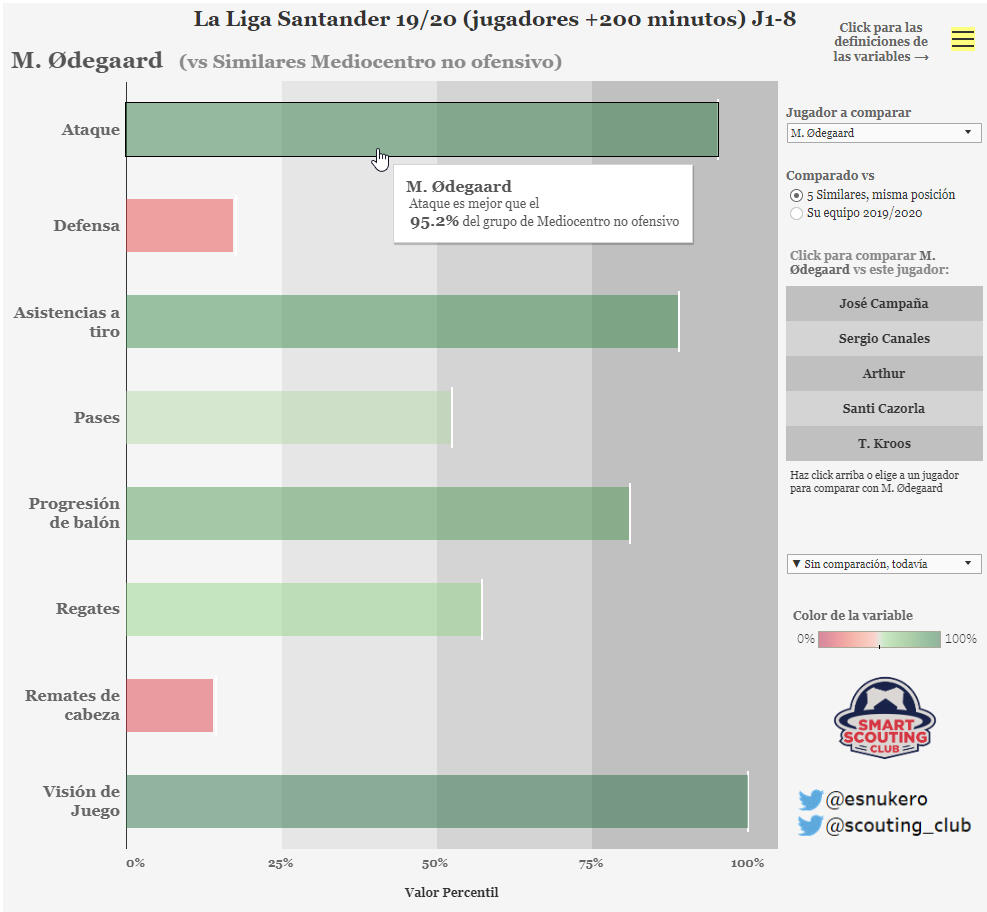
A primera vista se ven los valores del joven jugador noruego comparado con otros mediocentros de la liga. Destaca en los grupos que contienen métricas de ataque, asistencia de tiros, progresión de balón y visión de juego.
El panel gana importancia con los nombres que aparecen a la derecha, que son los 5 jugadores de su posición que tienen los números más similares a él. Si se hace click sobre uno de ellos se muestra la comparación en forma de línea azul sobre las barras. En este caso se destacan los nombres de José Campaña, Sergio Canales, Arthur, Santi Cazorla y Toni Cross.
Aquí la versión comparándolo con Santi Cazorla:
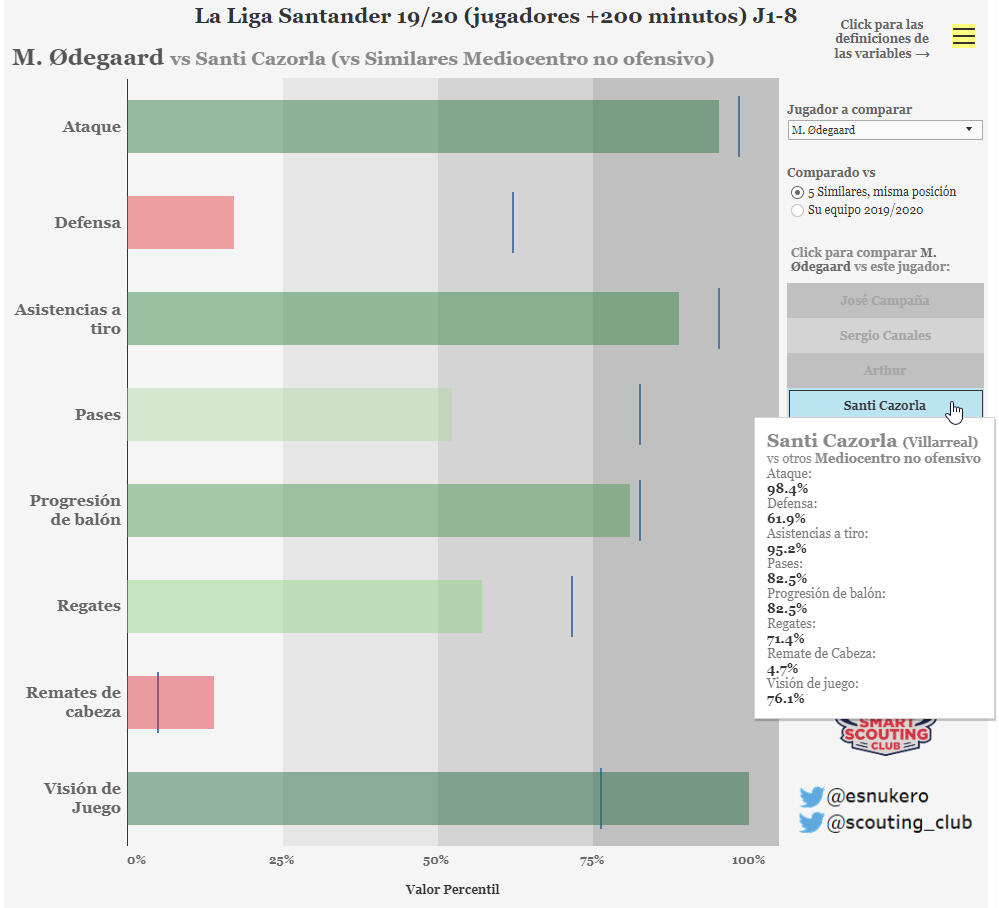
También podemos compararlo contra otro jugador de la lista total de jugadores, pero hay que tener en cuenta que, si no es de la misma posición, el percentil no será comparado con jugadores de la misma posición. Por ejemplo, si se compara con un delantero y tiene más defensa no quiere decir que sea mejor defendiendo, sino que, entre el grupo de delanteros, ese delantero tiene mejor defensa que Ødegaard frente a los jugadores de su posición.
Otra opción que ofrece es comparar al jugador principal con el resto del equipo (también teniendo en cuenta que cada uno está comparado con los jugadores de su posición):
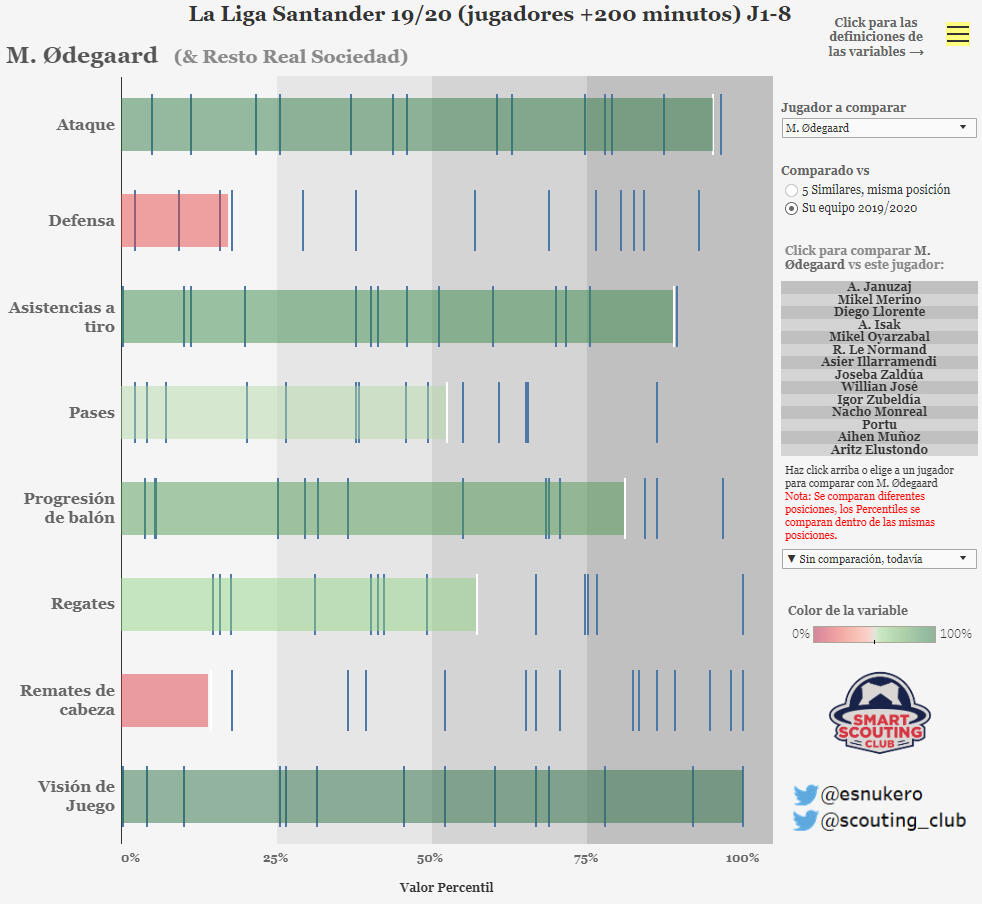
Clave en la construcción y en el ataque de su equipo, Ødegaard está siendo referente de la Real Sociedad en este inicio de liga.Este dashboard puede tener un gran poder de comparación ajustando los valores de las métricas, los grupos y las posiciones. Así como añadir otros factores como edad, altura, dar más valor a ciertas métricas si buscamos un jugador u otro… y también el añadir una liga o comparar jugadores de varias ligas juntos.
Las posibilidades son grandes y esto todavía no ha hecho nada más que empezar, pero seguro que este tipo de herramientas empiezan a ser cada vez más comunes en las secretarías técnicas de los equipos de fútbol (como ya pasa en la NBA de baloncesto) como apoyo e información adicional a sus informes.
Dashboard de Variables Liga SmartBank 19/20
He trabajado con datos de Instat para la creación de este Dashboard con filtros para seleccionar las variables de los ejes X e Y de #LaLigaSmartBank.
La selección de las variables de los ejes haced este panel una herramienta muy completa para combinar 2 métricas entre las más de 100 disponibles.
Otra opción es hacer el dashboard con los jugadores de la liga. Aquí con datos de Wyscout para la Segunda división española.
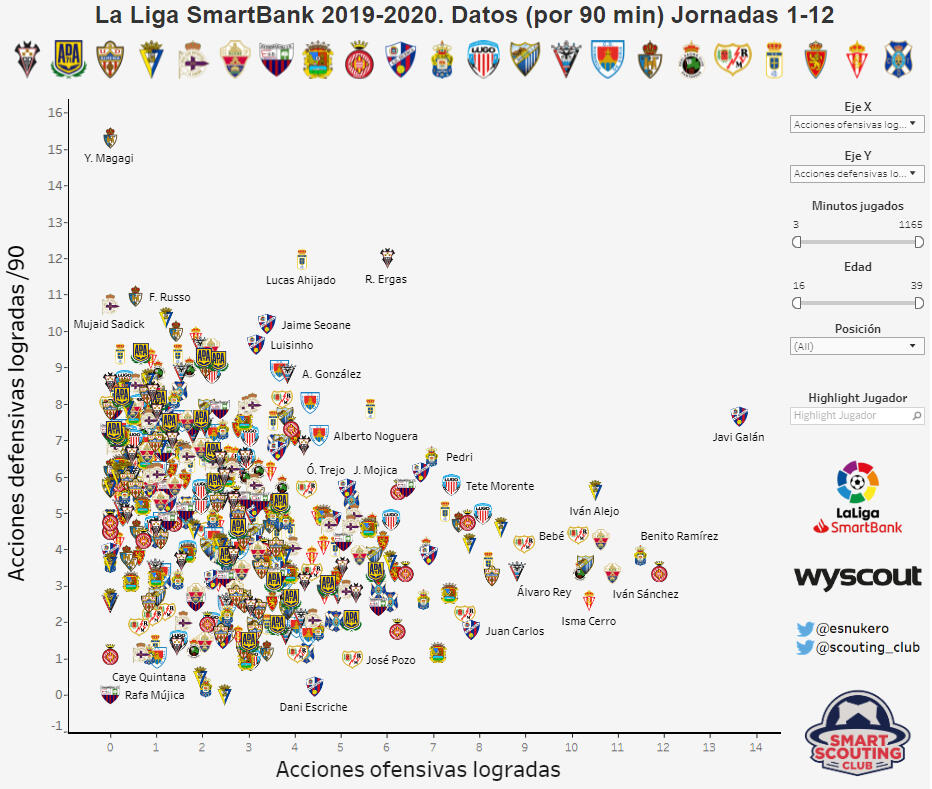
Alejandro Arribas. Fichaje del Real Oviedo para la temporada 19/20
#LaLigaSmartBank #Fichajes Alejandro Arribas llega desde México para reforzar la defensa central del @RealOviedo.
30 años, amplia experiencia en 1ª y gran relación con Michu y Egea
Para este gráfico ⬇️ he usado sus números durante el año 2018 y 2019 en México, vs La Segunda18/19
En este nuevo gráfico (a ver si os gusta y es claro!), el punto rojo es la posición de los números de Arribas en México comparado con el total de centrales de Segunda 18/19 (+1000 min jugados). La raya vertical es la media de todos, y las flechas los máximos y mínimos.
Lo que más destaca (y quizás lo más positivo para un central) es el % de duelos defensivos ganados (70%). Si, es otra liga, otro estilo... pero con ese número, hubiera sido el mejor de la temporada pasada en segunda, con un número medio de acciones defensivas.
Destacar también las pocas faltas cometidas, pese a ir a tope, parece que mide bien "el límite".
En el mapa de calor se ve que actuó (con balón) de central diestro, y también recibió balón más por detrás. Quizás pueda dar más opciones en defensa (como pasaba con Forlín).
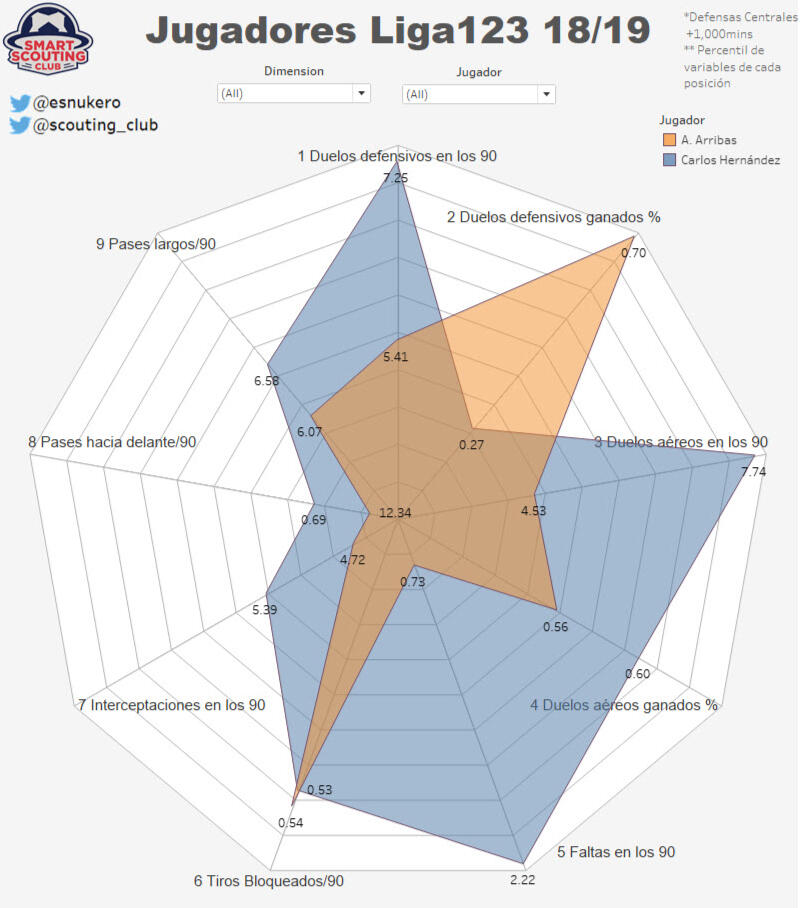
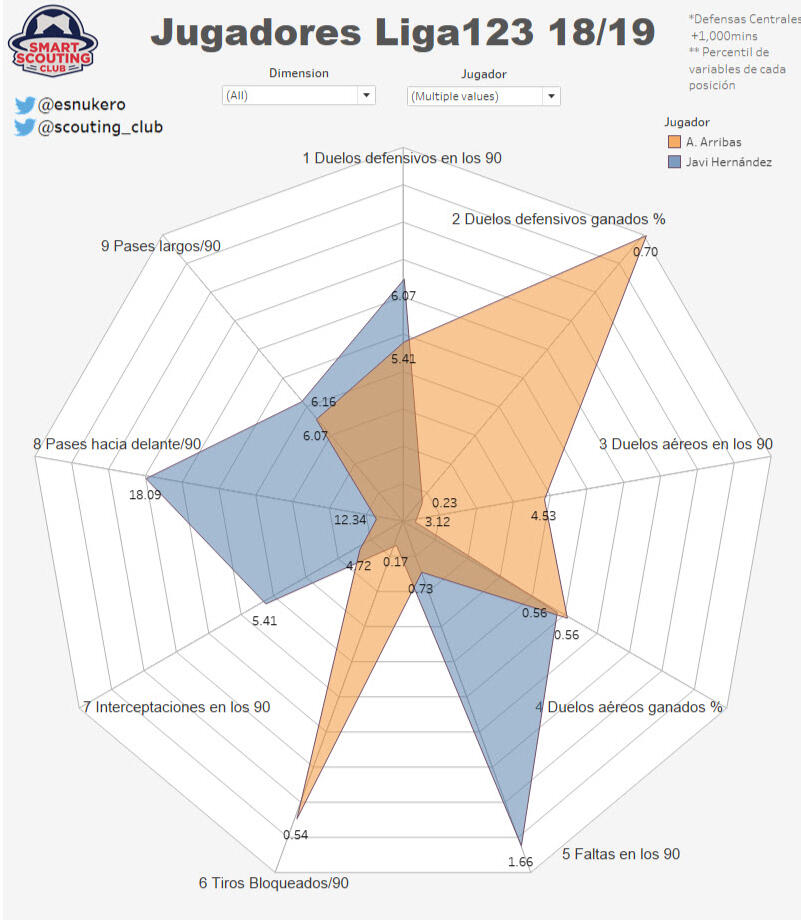
Un par de comparaciones con dos de los centrales de la temporada pasada, Javi Hernández y Carlos Hernández.
Carlos muchas más faltas y más acciones defensivas, pero con menos eficacia. Mejor en duelos aéreos. Similar en pases.
Con Javi mejor en defensa y menos pases.
Son ligas diferentes, pero estos son sus números en % de sus últimas 4 temporadas. Bastante regular, e incluso mejorando algunas. No tiene pinta que llegue "de retiro".
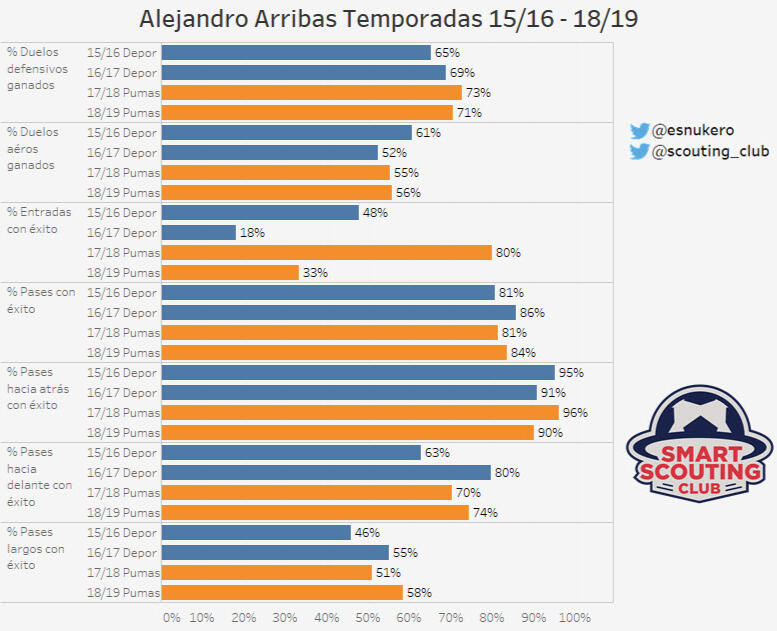
Visualización de datos para analizar estilos de juego (baloncesto)
Ayer la selección española femenina de baloncesto hizo historia ganando su cuarto Eurobasket tras derrotar a la selección de Francia en la final.Detrás de las jugadores y dentro del staff técnico está la zamorana Susana Ferreras, que actualmente trabaja como Data Scientist en el Arsenal de Unai Emery.En el baloncesto (y especialmente en la NBA), el uso de datos y su análisis para preparar partidos lleva siendo importante desde hace unos años.He aportado visualizaciones para sus artículos a la página web dedicada al análisis y scouting de baloncesto, Baskeroseno.com. Un ejemplo es esta gráfica en la que se comparan dos jugadores para conocer mejor el impacto en base a la frecuencia y la eficiencia según los diferentes tipos de jugadas.
El análisis es pertinente porque Andrew Albicy (azul) abandona Morabanc Andorra y Jeremy Senglin (verde) ha sido el fichaje que, presumiblemente, ocupará su rol de base titular. Esta posición es crucial para el rendimento de un equipo porque suele ser el director de juego y con mayor volumen de posesión de balón.Mostrar los tipos de jugada nos permiten entender de un vistazo el estilo de juego tanto individual como colectivo. En este caso, vemos rápidamente las diferencias y semejanzas entre ambos y qué puede aportar la llegada de Senglin y cómo afectará la salida de Albicy.Paralelamente, hemos hecho un estudio del estilo de juego de Andorra la temporada pasada que nos permitirá establecer una proyección de la frecuencia de cada tipo de jugada una vez se cierre la plantilla para la 2019/2020.El uso de estadísticas y datos ya está normalizado en la NBA y es cuestión de tiempo que se instaure de forma generalizada en Europa. Ya son muchos los equipos que utilizan big data para optimizar decisiones tácticas y afinar en los fichajes. Todo parece indicar que, más pronto que tarde, otros deportes seguirán la estela del fútbol y del baloncesto para incorporar los datos en su trabajo diario.
Segunda División 18/19. Mejores jugadores
Usando los datos de Wyscout, he realizado un estudio con un sencillo algoritmo para obtener dos equipos con los jugadores que más han destacado en la Segunda División Española en la temporada 2018/2019.
Podéis descargar el PDF completo con análisis jugador a jugador Aquí o en la imagen de abajo de los dos equipos obtenidos.
Top 6 European Leagues 18/19: NP Goals - NP xG / 90min vs NP Goals Scored/ 90min
Vemos en este dashboard la diferencia entre goles marcados (sin penaltis) y los goles esperados xG por 90 minutos jugados en las 6 Ligas más importantes de Europa (España, Inglaterra, Alemania, Italia, Francia y Portugal). Pudiendo filtrar por Liga, Posición, Minutos Jugados e incluso por nombre del jugador.- Messi promedió 1 gol por 90 minutos y estuvo por encima de sus expectativas, con 0.4 goles/ 90 min por encima de lo esperado (xG).
- Mbappé superó esas cifras de goles por partido promediando 1.16 por partido.
- Haris Seferović se cuela junto a Messi y Mbappé con 1 gol por partido y prácticamente mismos números que Messi.
- Christian Stuani, tras el descenso del Girona, será uno de los fichajes más deseados de este mercado de fichajes de verano para todo equipo que busque delantero centro. Promediando 0.4 goles por partido y en linea con sus números esperados.
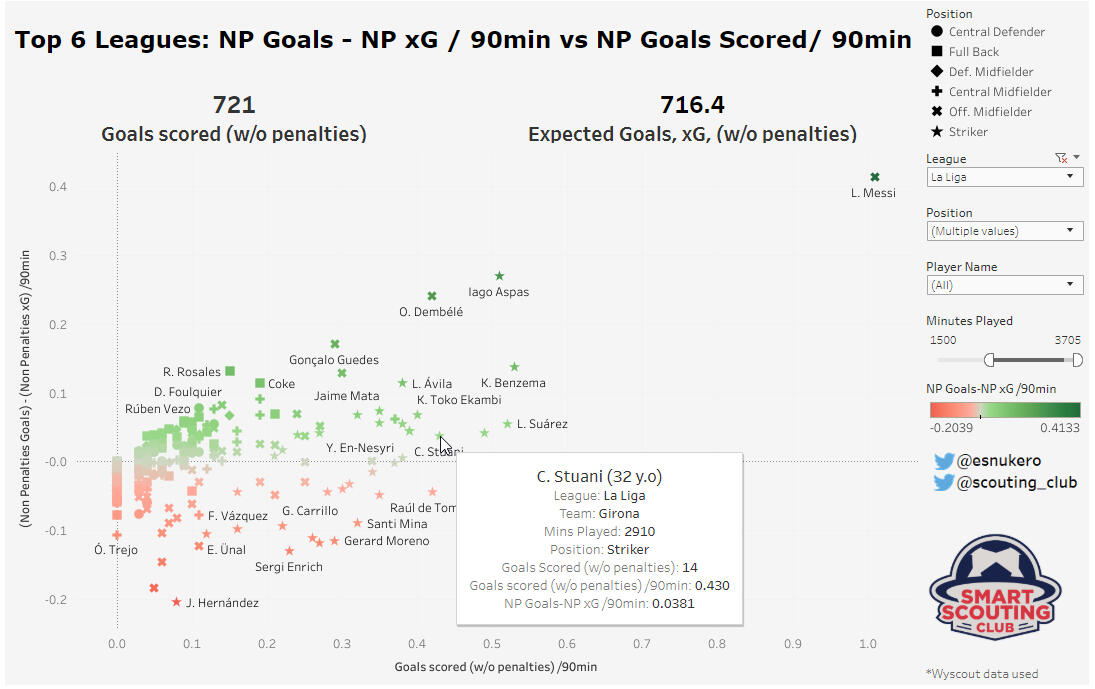
La Liga 18/19 Top 7: xG Contributors
#LaLiga #Datos Analizamos la contribución esperada de Goles (Sin penaltis) y Asistencias de los 7 Primeros equipos de la Liga 18/19.- Messi contribuyó en el 28% de los Goles Esperados (xG) y Asistencias Esperadas (xA) del #FCBarcelona. 29, dividido en 19 xG y 10 xA
#dataviz ⚽️📊
- En el #ATM, deberán sobreponerse a la marcha de #Griezmann, que contribuyó en el 23% de los xG y xA de su equipo con 9.4 Goles Esperados y 7 Asistencias Esperadas.
- En el #SevillaFC, ¿Seguirá Ben Yedder? Ha contribuido en un 21% de xG y xA del conjunto andaluz.
Fichajes Real oviedo - temporada 18/19
Sergio Tejera
Sergio Tejera se convirtió ayer en el primer fichaje del @RealOviedo. Voy a hacer un hilo con @scouting_club para analizar su temporada pasada y si, a priori, es buen fichaje. Primero, algunos de sus datos de la temporada 17/18 #dataviz #footballanalytics
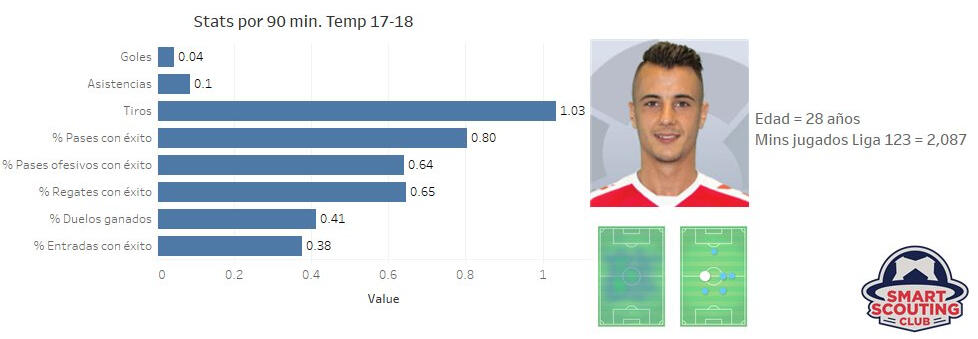
Mucho movimiento en el centro del campo, buen toque de balón con la zurda, y alto % de acierto en pases. Le faltó algún gol más. Lo vamos a comparar con otros jugadores de su posición (+/-) como Aleñá, Eguaras, Kunde, Michel, Iñigo Pérez... y con Rocha y Folch
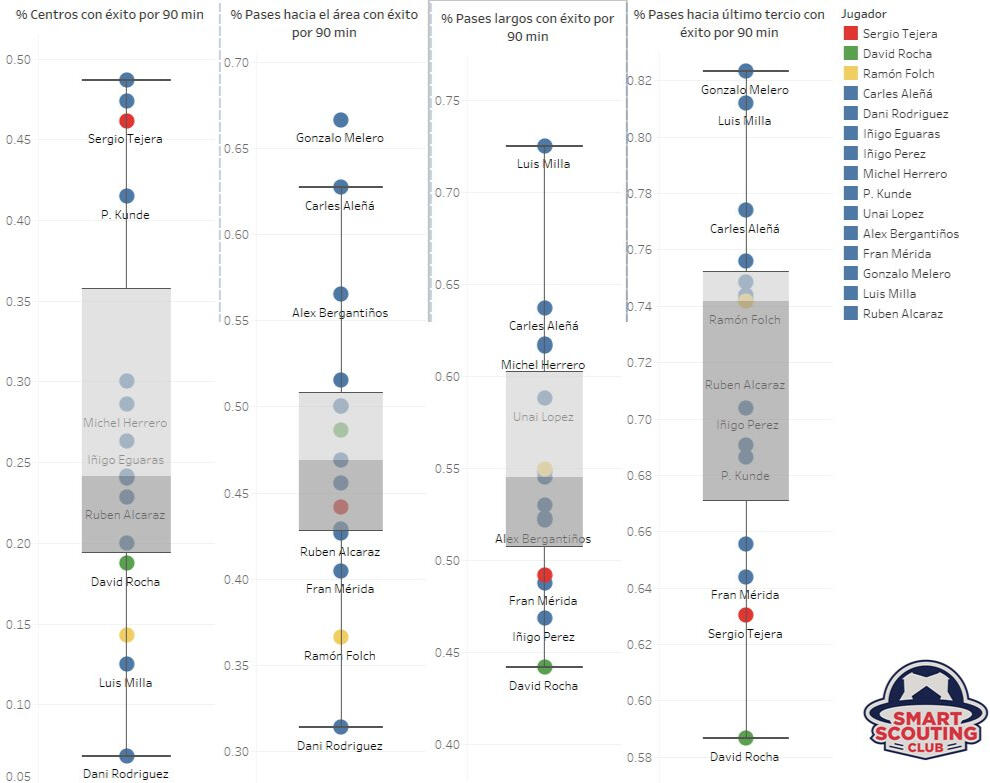
Tejera destaca aquí en su % de acierto en centros, y vemos como está en el medio entre Folch y Rocha. Aquí ocupará el puesto de creación que entre Rocha-Folch tuvieron este año, y lo vemos en esta imagen. Más volumen de pases recibidos x 90 min y pases interiores
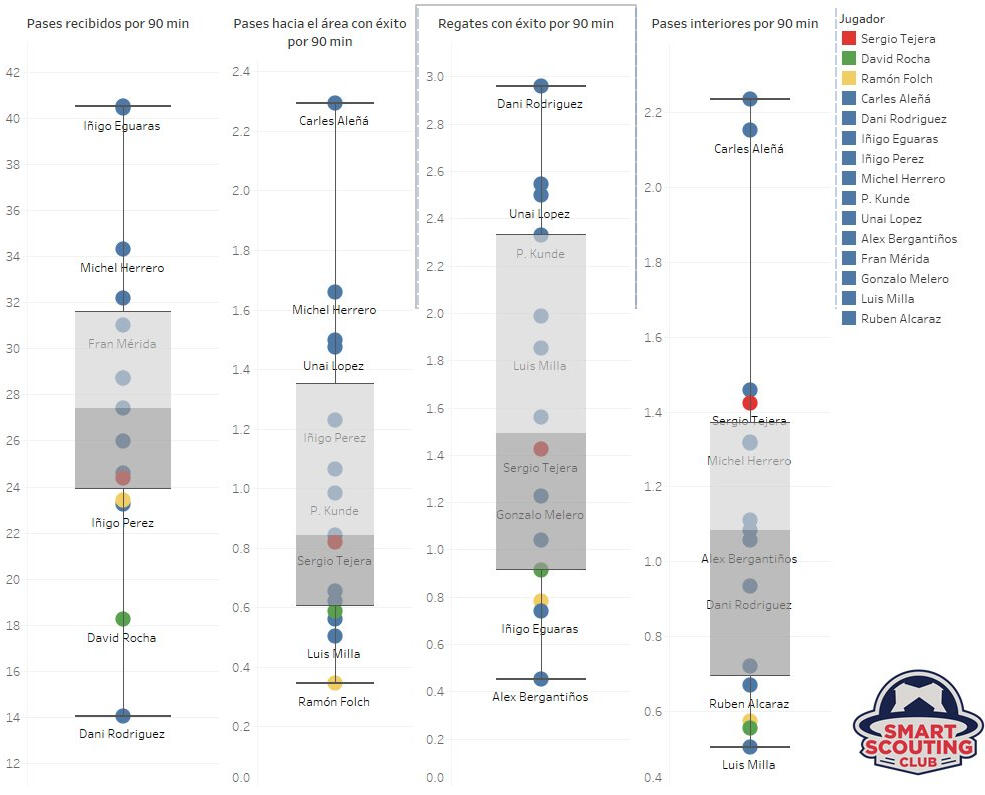
Los números de Tejera x 90 min se asemejan a Kunde o R.Alcaraz. Aportará esos pases entre líneas que tanto echó de menos el #RealOviedo esta temporada y que le vendrán de perlas.
Otro aspecto en el que Sergio Tejera destaca frente a Rocha/Folch es en el técnico, con los regates con éxito por 90 min, que le ayudan a sacar el balón. Sin duda esa zurda tiene mucha clase y hay que aprovecharla.
No es todo ofensivo, y Sergio Tejera es un jugador que se aplica en labores defensivas como recuperaciones y duelos defensivos/ofensivos (ambos por encima de Rocha y Folch). Además, al llevar el balón pegado, recibe muchas faltas (cerca de Aleñá en la gráfica de faltas x 90 min)
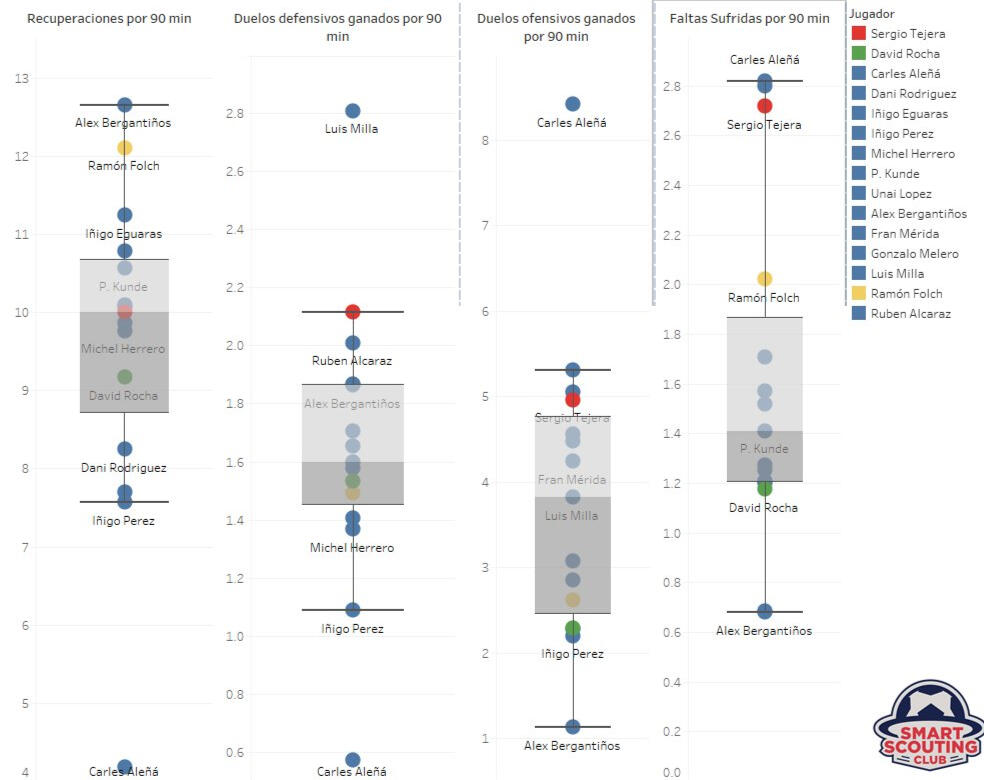
Los datos dicen que puede ser un jugador muy importante en el #RealOviedo, que llevará la batuta del centro del campo acompañado de Folch. Sólo esperar que le respeten las lesiones y pueda desplegar todo el fútbol que tiene esa zurda. #sportsdata #scouting #Liga123
Contact me!! Escríbeme!!
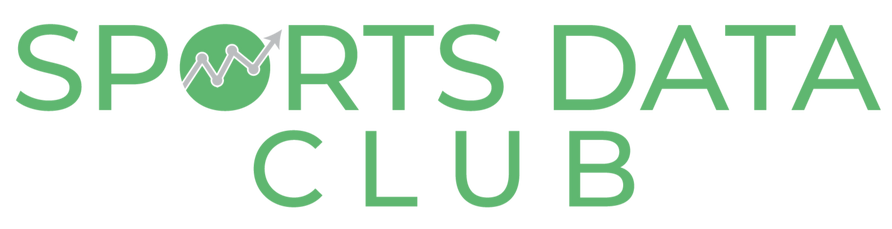
EN
If you want to contact me for samples, collaborations or other doubts, please fill the form below:
ES
Para contactar conmigo para alguna muestra, de cara a colaboraciones o trabajos, por favor rellene el siguiente formulario:
Thank you! Muchas Gracias!
EN
Thanks for your message, I will reply as soon as possible!ES
Muchas gracias por tu mensaje, responderé lo más rápido posible!